AI-based cytometer detects rare cells in blood using magnetic modulation and deep learning
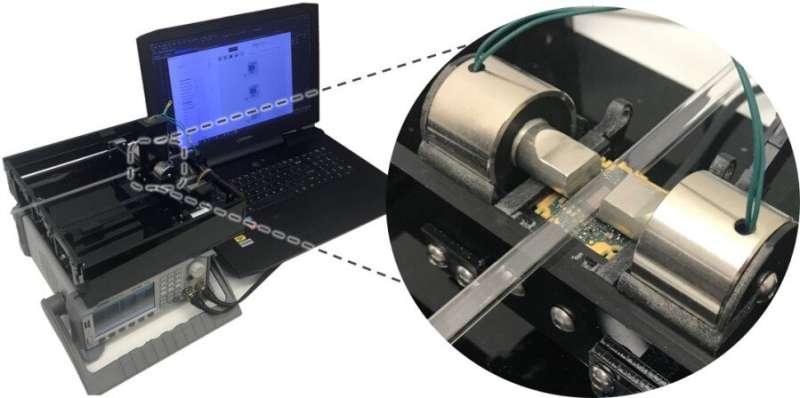
Detection of rare cells in blood and other bodily fluids has numerous important applications including diagnostics, monitoring disease progression and evaluating immune response. For example, detecting and collecting circulating tumour cells (CTCs) in blood can help cancer diagnostics, study their role in the metastatic cascade and predict patient outcomes. However, because each millilitre of whole blood contains billions of blood cells, the rare cells (such as CTCs) that occur at extremely low concentrations (typically lower than 100-1000 cells per millilitre) are very difficult to detect. Although various solutions have been developed to address this challenge, existing techniques in general are limited by high cost and low throughput.
Researchers at UCLA Henry Samueli School of Engineering have developed a new cytometry platform to detect rare cells in blood with high throughput and low cost. Published in Light: Science and Applications, this novel cytometry technique, termed magnetically modulated lensless speckle imaging, first uses magnetic bead labelling to enrich the target cells. Then the enriched liquid sample containing magnetic bead-labelled target cells is placed under an alternating magnetic field, which causes the target cells to oscillate laterally at a fixed frequency. At the same time, a laser diode illuminates the sample from above and an image sensor positioned below the sample captures a high-frame-rate lensless video of the time-varying optical pattern generated by the sample. The recorded spatiotemporal pattern contains the information needed to detect the oscillating target cells.
The researchers built a compact and low-cost prototype of this computational lensless cytometer using off-the-shelf image sensors, laser diodes and electromagnets, and used a custom-built translation stage to allow the imager unit to scan liquid sample loaded in a glass tube. The prototype can screen the equivalent of ~1.2 mL of whole blood sample in ~7 min, while costing only ~$750 and weighing ~2.1 kg. Multiple parallel imaging channels can also be easily added to the system to further increase sample throughput.
To ensure optimal sensitivity and specificity of rare cell detection, a two-step computational procedure was developed, which involved a computational motion analysis algorithm to detect micro-objects oscillating at the specified alternating frequency, and then a deep learning-based classification algorithm based on a densely connected pseudo-3-D convolutional neural network (P3D CNN) structure. The deep neural network greatly improved the accuracy of the technique, resulting in a limit of detection of 10 cells per millilitre of whole blood.
This AI-driven cytometry technique relies on the magnetic particles for both cell enrichment and detection, which reduces the time and cost for detecting rare cells while maintaining a high sensitivity. This compact, low-cost yet powerful cytometry system may find numerous applications especially in resource-limited settings.
More information: Yibo Zhang et al. Computational cytometer based on magnetically modulated coherent imaging and deep learning, Light: Science & Applications (2019). DOI: 10.1038/s41377-019-0203-5
This research was led by Dr. Aydogan Ozcan, Chancellor's Professor of Electrical and Computer Engineering at UCLA and an Associate Director of the California NanoSystems Institute (CNSI). The other authors of this work are Dr. Yibo Zhang, Dr. Mengxing Ouyang, Dr. Aniruddha Ray, Tairan Liu, Dr. Janay Kong, Bijie Bai, Dr. Donghyuk Kim, Alexander Guziak, Yi Luo, Alborz Feizi, Katherine Tsai, Zhuoran Duan, Xuewei Liu, Danny Kim, Chloe Cheung, Sener Yalcin, Dr. Hatice Ceylan Koydemir, Dr. Omai Garner and Dr. Dino Di Carlo. This work was supported by the KoƧ Group, NSF and HHMI.
Journal information: Light: Science & Applications